The landscape of Large Language Models (LLMs) is on the brink of a seismic shift. Here's a streamlined guide for AI and ML engineers to the pivotal changes on the horizon.
From Research to Reality
You Won't Believe How Cheaply You Can Train Your Own AI Model Now!
Forget the notion of GPT and LLaMa as mere products; they're the blueprint for the next generation of AI. The imminent clarity on architectures, pipelines, and datasets will slash training costs dramatically. Soon, pre-training a specialized model could cost you just $10-100k, even in a distributed setup. It's the democratization of AI development.
Inference on Steroids

Thanks to quantization, MoD, and optimization for edge devices, we're about to run 13-30 billion parameter models on our smartphones and other edge devices using ARM, TPU, and NPU architectures.The implications for application development and user engagement are staggering.
Fine-tuning Becomes Optional
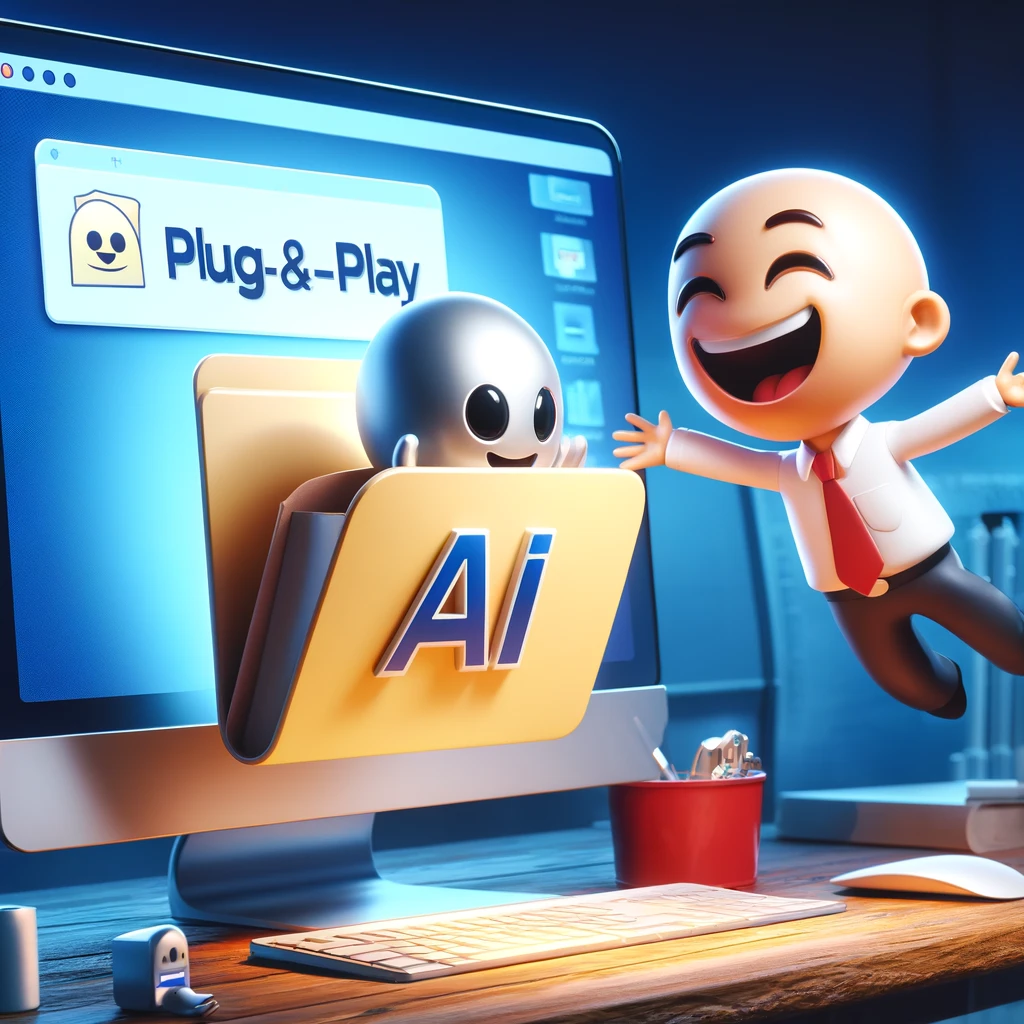
With models handling millions of tokens, the necessity for fine-tuning dwindles. Imagine feeding a model your entire organizational history in a single prompt and getting a bespoke model in return. The switch from one platform to another? It's about to become as easy as copy-paste. Imagine creating a personalized model by simply inputting a prompt encompassing 10-100 pages of your life's history or organizational records. This approach significantly lowers the barrier to obtaining tailored AI models, simplifying the transition between platforms like Zephyr and Hermes, or Cloud and Databricks, to mere clicks and copy-paste actions.
Adaptive Routing: The New Normal
The concept of adaptive routing is poised to redefine how applications interact with models and vice versa. This dynamic selection process, based on the specific requirements of a task and the current computational resource landscape, introduces an unprecedented level of flexibility and efficiency in model deployment and utilization.
The future is flexible: Applications will select models on-the-fly, tailored to specific tasks, while models choose their computational playground based on real-time hardware availability. This adaptive routing is set to revolutionize model deployment and efficiency. Example: "Martian" Router.
RAG Redefined
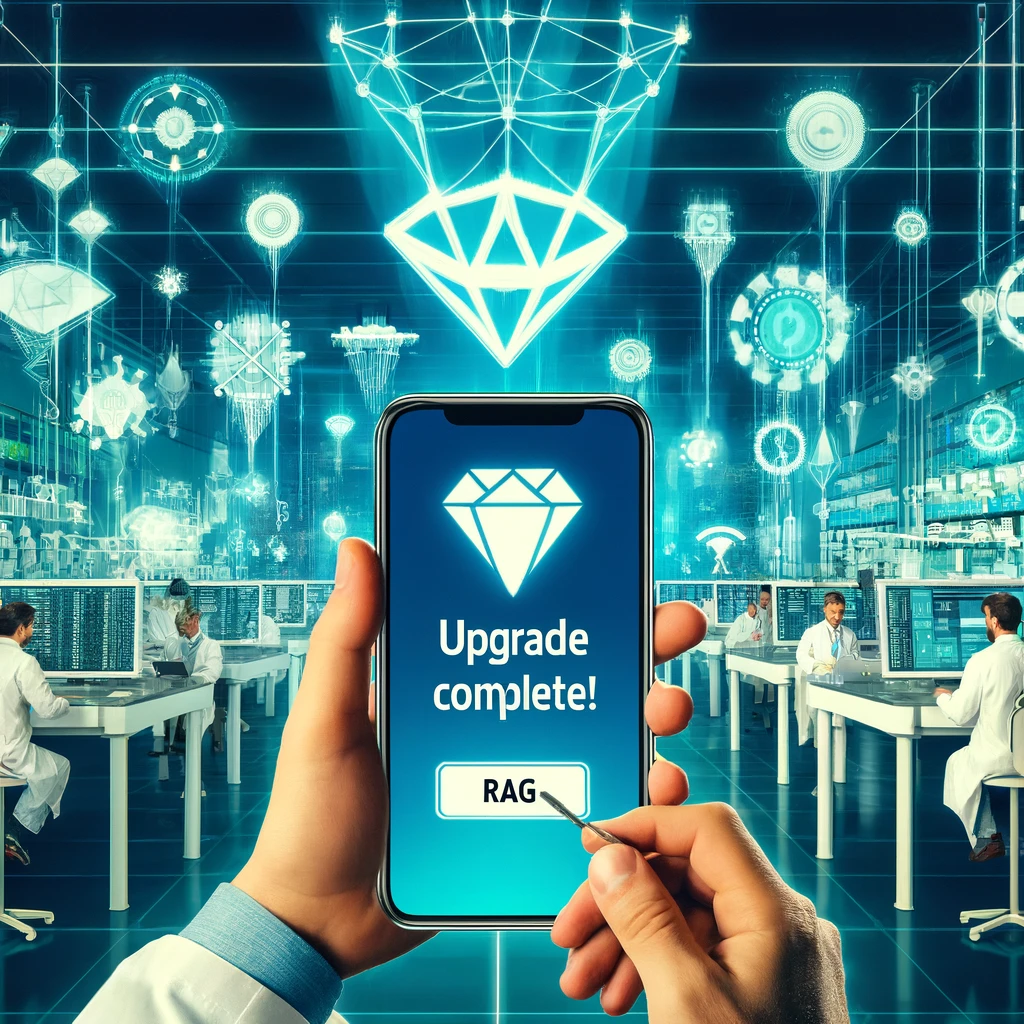
RAG isn't going anywhere; it's getting an upgrade. With decentralized datasets absorbing knowledge in real-time, base models will become leaner, faster, and yes, capable of running on a phone. This evolution signifies a partial shift away from traditional pre-training, paving the way for more agile and responsive models. Far from becoming obsolete, RAG is expected to partially replace the traditional pre-training process. The integration of vast, decentralized RAG datasets, encompassing billions or even trillions of tokens, will enable models to assimilate knowledge in real-time. This breakthrough will facilitate the development of base models that are not only leaner and faster but also capable of being deployed on "simple" devices.
For those at the cutting edge of AI and ML, these developments signal a period of unprecedented opportunity and innovation. The future of LLMs promises not just advancements in technology but a complete overhaul of how we approach, deploy, and interact with AI.